As the popularity and accessibility of Language Learning Models (LLMs) continue to grow among the general public, creative individuals are leveraging them to develop an extensive array of inventive applications.
In this post, we demonstrate how you can use the TitanML Platform to fine-tune your own LLMs for one such creative use-case: creating an app to detect the critical aspects of feedback and transform them into constructive, actionable and encouraging feedback.
This simple app would help people become more aware of how their words might affect others and give them examples on how to turn their feedback into constructive ones that would support healthy interpersonal relationships.
The Problem​
Critical vs. Constructive Feedback​
In order to give truly constructive feedback, it helps to understand the difference between constructive and critical feedback. Understanding this difference is crucial in turning feedback into a tool for learning and growth.
Critical feedback focuses on pointing out the problems without offering solutions. They can often be harsh, overly negative and directed at the qualities of a person rather than their work. They are often vague and can contain sweeping generalisations, as well as exaggerations, personal attacks and accusations. In addition, such feedback is usually emotionally charged and can often be demotivating and discouraging for the individual on the receiving end.
In contrast, constructive feedback is usually positive and uplifting with specific and actionable suggestions. The main aim of constructive feedback is to help the individual improve by objectively pointing out the strengths and weaknesses of the work done by the individual.
Preparation​
Here we are going to walk through the steps to fine-tuning your own LLMs, which you will be able to use in your application.
- Design the Model Specifications
- Generate datasets with OpenAI’s API
- Fine-tune models with TitanML
- Deployment with Titan Takeoff Server/Triton Server
Designing Model specifications​
The application we want to build would have two simple features:
- Identify instances of critical/constructive feedback and an explain why it is critical/constructive.
- Improve the given feedback by providing a constructive version of the feedback.
While both features could be implemented easily with an overarching LLM such as GPT-3 with different prompts, it may be far more efficient to use the two smaller models that are geared towards certain tasks.
For the first feature, we can use a sequence classification model that can produce a class label such as “critical” or “constructive” for each sentence. We could even go further to split the labels into more finer categories that would explain why the text is critical or constructive, such as the following:
- Positive Comment (constructive)
- Helpful Suggestion (constructive)
- Balanced Criticism (constructive)
- Vague Criticism (critical)
- Harsh Criticism (critical)
- Sarcastic Comment (critical)
- Blameful Accusation (critical)
- Personal Attack (critical)
- Threat (critical)
This is not meant to be an exhaustive list. There may be other categories of critical or constructive comments, or sentences that may fall into more than one category, but these categories would be more than helpful in identifying why a part of the feedback would be critical or constructive.
For the second feature, we can use an encoder-decoder model that would “translate” critical feedback into constructive feedback. One good model to use would be the T5 (Text to Text Transfer Transformer) model. The model would take in a string of text and produce another string of text.
Now that we’ve outlined the basic model to use, we can move on to finding datasets to fine-tune our models.
Generating datasets with OpenAI​
As there are no readily available labelled datasets relevant to our tasks, we will have to generate them with OpenAI’s API. This is a technique we have seen previously.
In order to train the two separate models, we have to generate one dataset for each model.
Dataset 1 (Classification dataset)
The first dataset will be used to train the sequence classification model, which will take in a piece of text and return its corresponding label. Thus, the dataset will require two columns: sentence and label. Here we are going to use OpenAI’s text-davinci-003 model as they are better at understanding more complex instructions and producing standardised outputs that will be easier to parse.
An example of the prompt we used to generate the dataset is as follows:
Details
Our prompt
You are an expert in providing constructive feedback and are conducting a workshop to teach people how to transform instances of negative feedback into constructive feedback. Critical feedback is usually vague, accusatory and often focuses on the negative qualities of a person without containing much details. Constructive feedback is uplifting, given with a compassionate and helpful attitude, and usually contains clear and actionable suggestions for improvement. Can you generate 10 examples of critical feedback that contains harsh criticism (this can be replaced with labels from other categories) ?This should be the format of the json:
[
"Your work lacks the quality to meet the requirements.",
"You seem clueless when it comes to executing this task."
]
Dataset 2 (Translation Dataset)
The second dataset will be used to train the T5 model, which will take in a text containing a critical feedback and return the constructive version of the same feedback.
While generating the datasets for the first time, we discovered that most of the critical feedback we generated pertained to presentations (e.g. your presentation lacked a sense of structure, your presentation was boring etc.). This was probably because the example feedback we gave to the prompt was about presentations. This instance of oversampling can lead to poor performance of the model with real-world data. In order to address this, we specifically requested these examples of feedback to be from a different workplace context.
We used the following improved prompt:
Details
Our improved prompt
You are an expert in providing constructive feedback and are conducting a workshop to teach people how to transform instances of negative feedback into constructive feedback. Negative feedback is usually vague, accusatory and often focuses on the negative qualities of a person without containing much details. Constructive feedback is uplifting, given with a compassionate and helpful attitude, and usually contains clear and actionable suggestions for improvement.Here is an example:
Negative Feedback: “Why was your presentation so confusing? You know that not everyone thinks like you.”
Constructive Feedback: “I think your presentation was ambitious in terms of coverage but could have been structured better to help audience to follow your presentation better. Would you be able to restructure your presentation the next time?”
Can you generate 5 pairs of negative feedback and the constructive version of each feedback in a different workplace context and put it in json format?
This should be the format of the json:
[
{
"Context": "You are a manager at a consulting firm and you are giving feedback to a junior consultant on their report.",
"Negative Feedback": "You are spending too much time on meaningless tasks.",
"Constructive Feedback": "I think you are doing a great job formatting the report and designing the charts, however, it would be great if you could first focus on getting the research to a good standard first."
},
{
"Context": "You are the portfolio manager of a hedge fund and you are giving feedback to an analyst on their stock pitch.",
"Negative Feedback": "Why didn't you include the fundamentals of the company in your report?",
"Constructive Feedback": "I liked how concise your report was in summarizing the main points, but the clients might demand a bit more research on the fundamentals of each stock. Could you include more information in your next version?"
}
]
Dataset Preparation​
We used JSON as a default output format for OpenAI as it is relatively standardised and easy to parse. We then converted the data and compiled them into a single csv file. Afterwards, we shuffled the rows of the dataset to ensure the labels are distributed evenly throughout, before splitting the dataset into two files, train.csv and validation.
For the Translation dataset, we combined the context column and negative feedback column into a single column as an instruction.
{
"Context": "You are a manager at a consulting firm and you are giving feedback to a junior consultant on their report",
"Negative Feedback": "You are spending too much time on meaningless tasks"
}
becomes:
"Context: You are a manager at a consulting firm and you are giving feedback to a junior consultant on their report. Make the following feedback constructive: You are spending too much time on meaningless tasks."
Fine-tuning models with TitanML​
Now that we have generated our datasets with OpenAI, we can use TitanML to fine-tune models for our specific tasks.
First, we uploaded the classification dataset to TitanHub with the Iris CLI:
iris upload <dataset_directory_path> feedback_classification_dataset
Next, we used the command generator feature to dispatch a new job/experiment. For this fine-tuning experiment, we are using the google/electra-base-discriminator model from HuggingFace. We can also select the dataset we previously uploaded with the dropdown.
As this is a sequence classification model, we had to fill in the number of labels and text field. We also have the option to provide configurations for hyperparameter tuning.
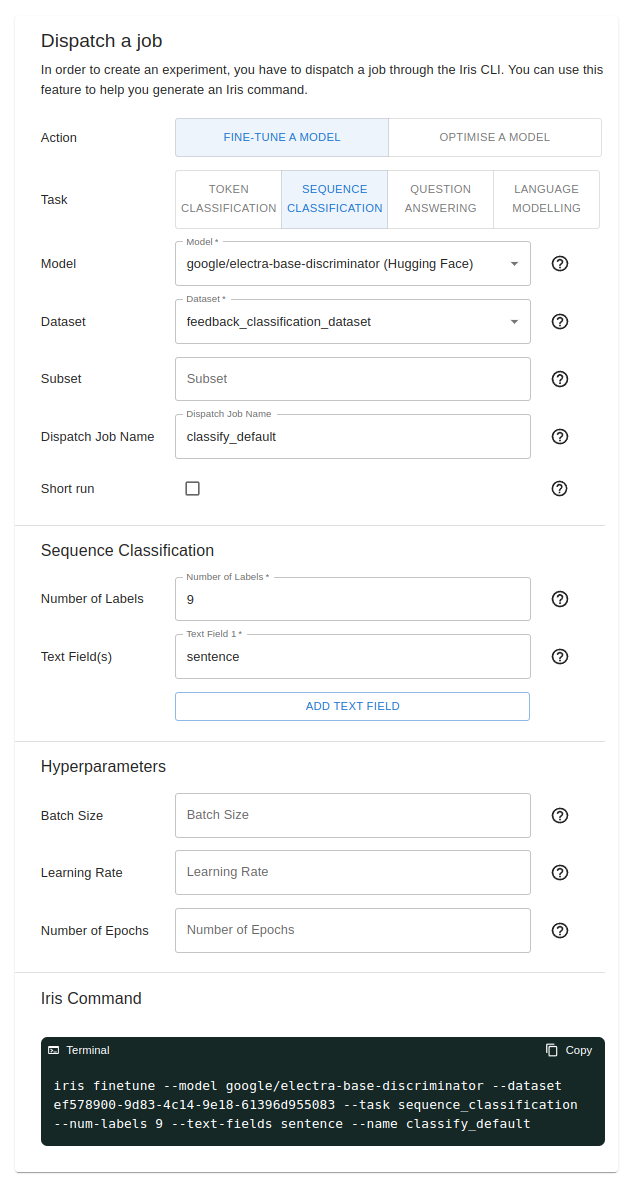
We can then run the Iris command from the terminal to dispatch a fine-tuning experiment to our cluster. Alternatively, you may also try out the one-click dispatch feature that’s available if your models and datasets are already on HuggingFace or uploaded to Titan Hub.
After running four different fine-tuning experiments, with a different number of epochs (1, 2, 3 and 4), we found that the model trained for 3 epochs give the optimal results (highest accuracy, lowest loss). We will use this model for our application to classify feedback.
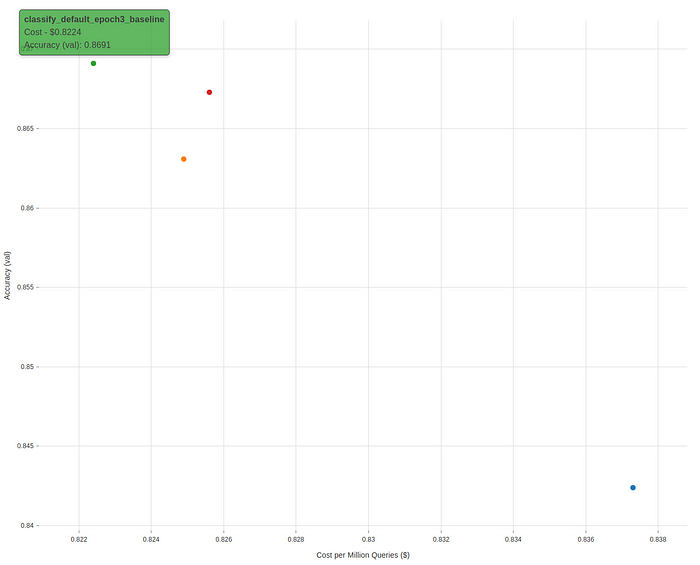
For the T5 Model, the process is quite similar: we upload the dataset, then use the command generator to generate an Iris command to dispatch our experiment. However, in terms of evaluating the performance of models, it may be better to use the inference API by evaluating their outputs. This time, instead of focusing on hyperparameter tuning, we can try fine-tuning different variants of Google’s Flan T5 Model (Flan-T5-Small, Flan-T5-Large, Flan-T5-XL) to see which model gives us the best performance/size trade-off.
For generative models, we can use the Titan Inference API to test each models by giving them an input and judging them by the quality of their outputs.
We gave the models the following inputs to be made constructive:
Inputs
Context: You are a manager giving feedback to your subordinate, who has been underperforming severely over the past few months.
Critical text: Your performance over the past few months has been absolutely disappointing. It doesn’t seem that you’ve put in any effort in improving your performance at all. I’m afraid that we will have to evaluate your position in this company if this continues.
These are the outputs of the fine-tuned models:
Outputs
Flan-T5-small: I would suggest that your subordinate acted independently as he/she has been underperforming in the past. Please let me know if this is remedied.
Flan-T5-large: I hope this criticism shows that you’ve put your efforts in. Keep up the good job of managing your performance.
Flan-T5-XL: I think that you have tried your best to improve your performance, however, it would be beneficial for you to focus on your own strengths and learn from your mistakes to help improve your performance. Would you be able to set some time aside to focus on your own strengths and improve your performance?
From these examples, we see that the Flan-T5-small and Flan-T5-large models seem confused about the task, responding to the feedback rather than transforming it into a constructive version. Only the fine-tuned Flan-T5-XL model was able to produce coherent responses and thus this is what we will use for our application.
Deploying the models​
In order to inference these models on-demand, we would need to deploy them. These are the two main ways to do them within the Titan ecosystem.
Titan Takeoff Server​
For our T5-Model, one of the most convenient options is to use the Titan Takeoff Server, accessible through our Iris CLI. You can find detailed instructions on how to do this here. Apart from a built-in CLI chatbot, you will also be able to spin up a server and make API requests to it, receiving either complete responses or streaming token by token.
The finished product​
After deploying our models, we can then use the associated endpoints to inference our models. We have built a simple frontend where users can input their feedback and provide the context of their feedback. Upon submission, each sentence would be analysed for its constructiveness and the user will be given a chance to improve the feedback with the click of a button. With a few simple steps, users will be able to get a simple analysis of their feedback and an improved version of their feedback.
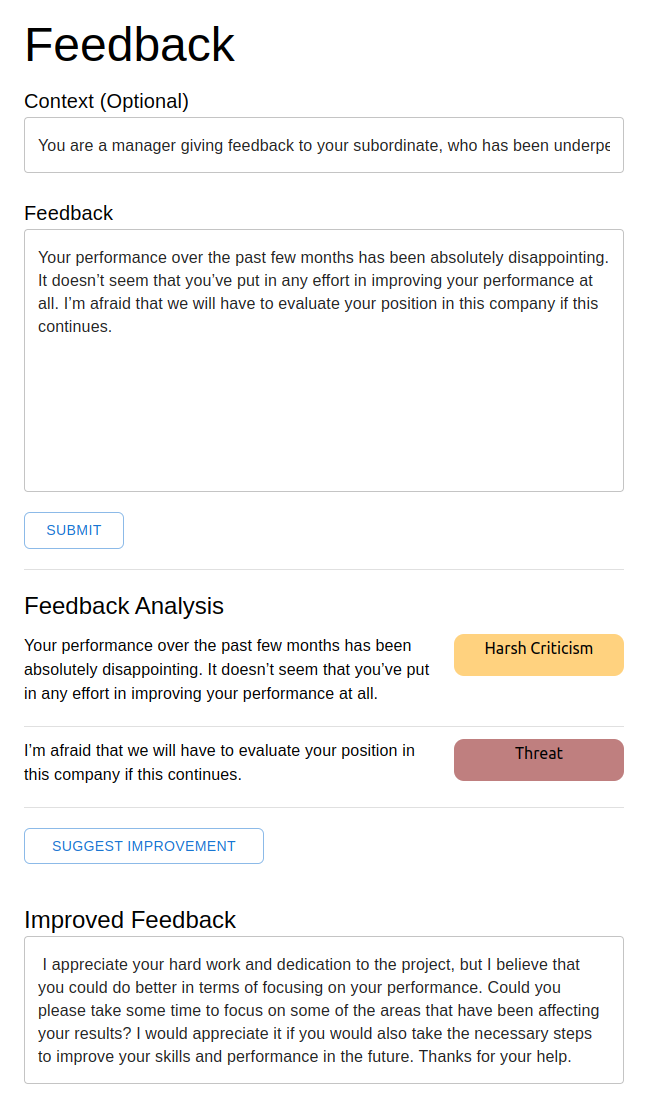
Conclusion​
With the TitanML Platform, you can fine-tune LLMs easily for almost every use-case, and with the Titan Takeoff Inference Server, you can deploy them in production with ease. To start applying cutting edge ML performance and latency optimisations to your own projects and models, checkout the TitanML platform! If you have any questions, comments, or feedback on the TitanML platform, please reach out to us on our discord server. For help with LLM deployment in general, or to signup for the pro version of the Titan Takeoff Inference Server, with features like automatic batching, multi-gpu inference, monitoring, authorization, and more, please reach out at hello@titanml.co.